Deep Learning for Symbolic Mathematics: A Game-Changer for Small Businesses in AI?
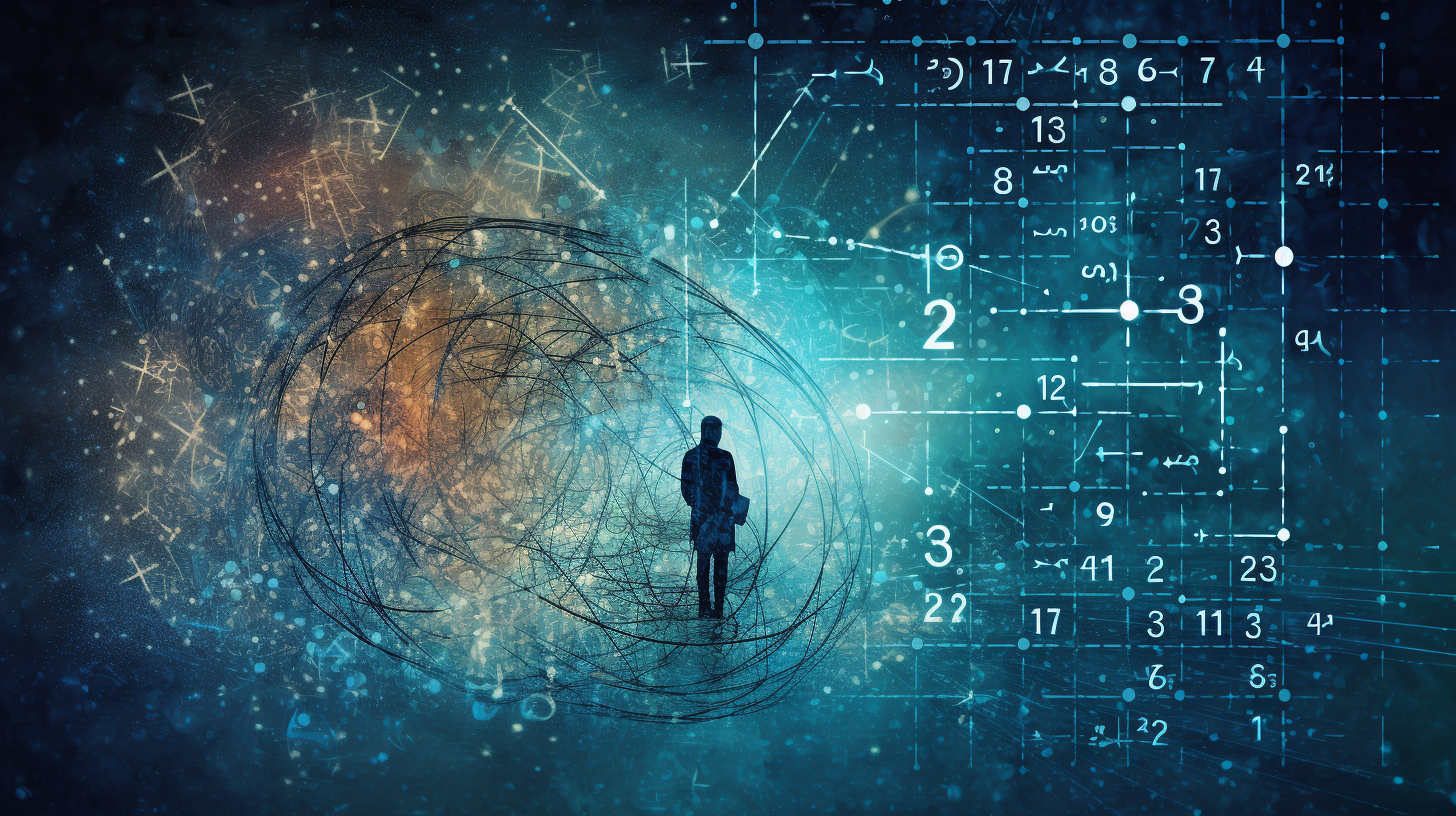
In a world where artificial intelligence (AI) is increasingly becoming a part of our daily lives, a recent scientific paper titled “Deep Learning for Symbolic Mathematics” has made a significant splash in the AI community. The paper, authored by a team of researchers, discusses the development of a deep learning model capable of solving complex mathematical problems, a task traditionally handled by symbolic mathematics software.
The researchers trained a Transformer model, a type of deep learning model, on a dataset of millions of mathematical expressions. The model was trained to predict the next token in a sequence, similar to how language models are trained. The results were nothing short of impressive. The model was able to solve complex integration problems and differential equations that are challenging for traditional symbolic mathematics software.
A New Era for AI in Mathematics?
This development raises an intriguing question: Could building instructive models be easier than we were led to believe? The paper’s findings suggest that this might be the case. The model’s ability to generalize and solve problems that were not in its training set demonstrates its potential for real-world applications. This could mean that AI models might not need to be as specialized as we previously thought, opening up new possibilities for their use in various fields.
Implications for Small Businesses and Solopreneurs
For small businesses and solopreneurs working in the AI field, this development could be a game-changer. The ability to use AI to solve complex mathematical problems could open up new avenues for innovation and efficiency. For instance, businesses could use these models to optimize their operations, make better predictions, or even develop new products and services.
Moreover, the potential ease of building such models could lower the barrier to entry in the AI field. This could enable more small businesses and solopreneurs to leverage the power of AI, leading to a more diverse and innovative AI landscape.
Looking Ahead
While the model developed in this paper is powerful, the authors note that it is not perfect and sometimes makes mistakes. This honesty about the model’s limitations is refreshing and highlights the need for further research and development in this field.
As we move forward, it will be interesting to see how these findings influence the AI field. Will we see more businesses adopting this technology? And how will this impact the way we approach problem-solving in mathematics and beyond? Only time will tell, but one thing is clear: the future of AI looks brighter than ever.